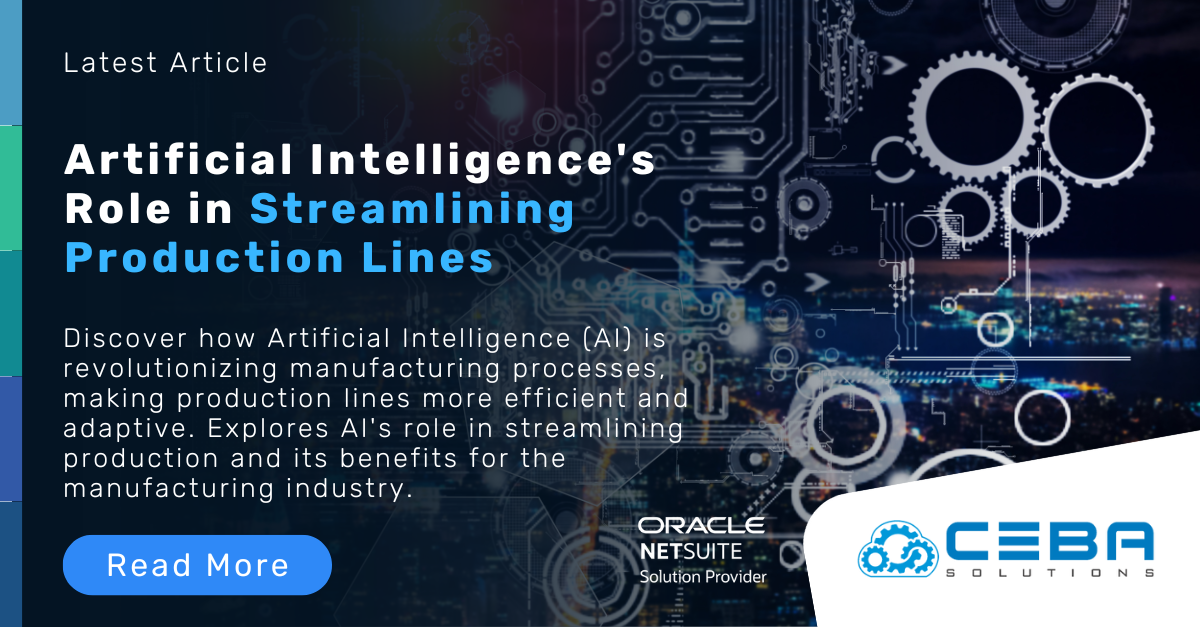
Artificial Intelligence's Role in Streamlining Production Lines
Artificial Intelligence's Role in Streamlining Production Lines
In the modern manufacturing landscape, efficiency and productivity are paramount. With the increasing complexity of production processes and the demand for higher quality products, traditional manufacturing methods are often insufficient to meet these challenges. Artificial intelligence (AI) is revolutionizing the way production lines operate, offering advanced solutions to enhance efficiency, reduce waste, and improve product quality. This article explores the role of AI in streamlining production lines, the benefits it brings, and key implementation strategies.
The Impact of AI on Production Lines
AI integrates machine learning, robotics, computer vision, and big data analytics to optimize various aspects of production lines. Here are some of the key ways AI is transforming manufacturing processes:
Predictive Maintenance
Anomaly Detection: AI algorithms analyze data from sensors and equipment to detect anomalies that may indicate potential failures. By identifying these issues early, maintenance can be scheduled proactively, reducing downtime and extending equipment life. This proactive approach not only minimizes unexpected breakdowns but also ensures that machinery operates at peak efficiency, thus extending the lifespan of the equipment and maintaining a consistent production flow.
Real-Time Monitoring: Continuous monitoring of equipment performance helps ensure that machines operate optimally. AI systems can predict when a machine is likely to fail and recommend maintenance actions, preventing unexpected breakdowns. This real-time insight allows for immediate corrective measures, enhancing overall productivity. The ability to foresee potential issues and address them before they become critical significantly reduces unplanned downtime and maintenance costs.
Quality Control and Inspection
Computer Vision: AI-powered computer vision systems can inspect products for defects with high precision. These systems use cameras and image processing algorithms to detect even the smallest imperfections that might be missed by human inspectors. This high level of accuracy ensures that only quality products reach the market. The integration of computer vision in quality control not only improves product reliability but also speeds up the inspection process, thereby increasing overall throughput.
Automated Quality Assurance: AI automates quality assurance processes by analyzing production data in real-time. This helps in identifying trends and patterns that could lead to defects, allowing for immediate corrective actions. Automated quality checks improve consistency and reduce the risk of defective products. By continuously monitoring and analyzing data, AI-driven quality assurance systems can quickly adapt to changes in production conditions, ensuring that quality standards are consistently met.
Process Optimization
Production Scheduling: AI optimizes production schedules by analyzing various factors such as order volume, machine availability, and labor resources. This ensures that production is balanced and resources are utilized efficiently. Optimized scheduling reduces idle times and maximizes output, enabling manufacturers to meet tight deadlines and adjust to fluctuating demand without compromising on efficiency.
Supply Chain Management: AI enhances supply chain efficiency by predicting demand, optimizing inventory levels, and coordinating with suppliers. This reduces lead times and ensures that materials are available when needed, preventing delays and bottlenecks in the production process. By providing real-time insights and forecasts, AI helps manufacturers maintain a smooth flow of materials and components, which is critical for just-in-time production environments.
Robotics and Automation
Robotic Process Automation (RPA): AI-powered robots can perform repetitive tasks with high accuracy and speed, reducing the need for manual labor. This not only increases productivity but also minimizes human error, leading to more consistent quality. The use of RPA in manufacturing allows human workers to focus on more complex and value-added tasks, enhancing overall operational efficiency.
Collaborative Robots (Cobots): Cobots work alongside human workers, assisting with tasks that require precision and strength. AI enables these robots to adapt to various tasks and work safely with humans. This collaboration enhances efficiency and allows human workers to focus on more complex tasks. By integrating cobots into the production line, manufacturers can achieve a flexible and scalable workforce that can quickly respond to changing production needs.
Energy Management
Energy Consumption Optimization: AI systems analyze energy usage patterns and identify opportunities to reduce consumption. By optimizing machine operations and production schedules, manufacturers can lower energy costs and reduce their environmental impact. This not only cuts costs but also supports sustainability goals. AI-driven energy management solutions help manufacturers meet regulatory requirements and enhance their reputation as environmentally responsible businesses.
Benefits of AI in Production Lines
Increased Efficiency and Productivity
Optimized Processes: AI optimizes production processes by identifying inefficiencies and recommending improvements. This leads to faster production cycles and higher output, maximizing the use of available resources. The continuous improvement driven by AI ensures that production lines remain agile and capable of adapting to new challenges and opportunities.
Reduced Downtime: Predictive maintenance and real-time monitoring minimize unplanned downtime, ensuring continuous production and higher equipment utilization. This proactive approach keeps production lines running smoothly. By anticipating maintenance needs and preventing breakdowns, AI helps manufacturers avoid costly disruptions and maintain consistent production schedules.
Enhanced Quality and Consistency
Consistent Quality: AI-driven quality control ensures that products meet high-quality standards consistently, reducing the rate of defects and rework. This consistency builds customer trust and satisfaction. High-quality products lead to fewer returns and complaints, enhancing the brand's reputation and customer loyalty.
Accurate Inspections: Automated inspections powered by AI are more accurate and reliable than manual inspections, ensuring that only high-quality products reach the market. This reduces returns and complaints, enhancing the brand's reputation. By leveraging AI for quality control, manufacturers can maintain rigorous standards while increasing inspection speed and efficiency.
Cost Savings
Lower Operational Costs: By automating tasks and optimizing resource usage, AI reduces labor costs, material waste, and energy consumption. These efficiencies translate into significant cost savings. The cost reductions achieved through AI-driven automation and optimization can be reinvested in further innovation and growth initiatives.
Efficient Resource Utilization: AI ensures that resources such as raw materials, labor, and equipment are used efficiently, minimizing waste and maximizing productivity. This optimization enhances overall profitability. By making better use of available resources, manufacturers can improve their bottom line while maintaining high standards of quality and efficiency.
Improved Decision Making
Data-Driven Insights: AI provides actionable insights based on comprehensive data analysis, enabling informed decision-making. Manufacturers can identify trends, predict future outcomes, and make strategic decisions with greater confidence. Data-driven decision-making helps manufacturers stay competitive by quickly adapting to market changes and seizing new opportunities.
Proactive Problem Solving: AI's ability to predict and prevent issues before they occur allows manufacturers to address potential problems proactively, avoiding costly disruptions. This foresight improves overall operational resilience. By identifying potential challenges early, manufacturers can implement corrective measures and maintain smooth operations.
Implementing AI in Production Lines
Assessment and Planning
Needs Assessment: Conduct a thorough assessment of the production line to identify areas where AI can add the most value. Consider factors such as production bottlenecks, quality control issues, and maintenance challenges. A comprehensive needs assessment helps prioritize AI implementation efforts and ensures that resources are allocated effectively.
Strategic Planning: Develop a strategic plan for AI implementation, outlining goals, timelines, and resource requirements. Ensure alignment with overall business objectives and stakeholder buy-in. A well-defined plan provides a clear roadmap for AI integration and helps secure the support needed for successful implementation.
Data Collection and Integration
Comprehensive Data Collection: Collect data from various sources, including sensors, machines, and production logs. High-quality data is essential for training AI models and making accurate predictions. Ensuring data accuracy and completeness is critical for the effectiveness of AI-driven solutions.
System Integration: Integrate AI systems with existing manufacturing execution systems (MES), enterprise resource planning (ERP) systems, and other relevant platforms to ensure seamless data flow and process coordination. Effective integration enhances the functionality of AI systems and enables real-time decision-making.
AI Model Development and Training
Algorithm Selection: Choose appropriate machine learning algorithms for specific tasks, such as predictive maintenance, quality control, and process optimization. Common algorithms include neural networks, decision trees, and support vector machines. Selecting the right algorithms is crucial for achieving accurate and reliable results.
Model Training: Train AI models using historical data and continuously update them with new data to improve accuracy and adaptability. Ongoing training ensures that AI models remain effective and responsive to changing production conditions.
Implementation and Scaling
Pilot Projects: Start with pilot projects to test AI applications on a small scale. Evaluate the results and make necessary adjustments before scaling up. Pilot projects provide valuable insights and help identify potential challenges before full-scale deployment.
Full-Scale Deployment: Once pilot projects are successful, roll out AI applications across the entire production line. Monitor performance and make continuous improvements. Scaling AI solutions requires careful planning and ongoing support to ensure optimal performance.
Employee Training and Support
Training Programs: Develop training programs to help employees understand and effectively use AI tools. Emphasize the importance of data-driven decision-making and the benefits of AI. Comprehensive training ensures that employees are equipped to leverage AI technologies effectively.
Ongoing Support: Provide ongoing technical support and resources to ensure that employees can troubleshoot issues and optimize AI systems. Continuous support helps maintain the effectiveness of AI solutions and encourages employee engagement.
Challenges and Considerations
Data Privacy and Security
Data Protection: Implement robust data security measures to protect sensitive manufacturing data from unauthorized access and breaches. Ensuring data security is critical for maintaining trust and compliance with regulatory requirements.
Compliance: Ensure compliance with industry regulations and standards regarding data privacy and security. Adhering to legal and regulatory requirements helps avoid potential legal issues and ensures the integrity of data management practices.
Initial Costs and ROI
Upfront Investment: Be prepared for the initial costs associated with implementing AI solutions, including hardware, software, and training. Initial investment is necessary for long-term benefits and should be carefully planned and justified.
Long-Term ROI: Focus on the long-term return on investment, considering the cost savings, efficiency gains, and quality improvements that AI can deliver. Long-term ROI should guide decision-making and investment strategies.
Change Management
Adoption and Acceptance: Foster a culture of innovation and encourage acceptance of AI-driven solutions among employees. Highlight the benefits and provide support during the transition. Effective change management is crucial for successful AI implementation and employee buy-in.
Conclusion
AI is revolutionizing production lines by enhancing efficiency, improving quality, and reducing costs. By leveraging advanced technologies such as predictive maintenance, computer vision, process optimization, robotics, and energy management, manufacturers can achieve significant improvements in productivity and operational performance. Implementing AI in production lines requires careful planning, data integration, and employee training, but the benefits far outweigh the challenges. As AI technology continues to evolve, its impact on manufacturing will only grow, driving innovation and excellence in the industry. Embracing AI is not just a competitive advantage but a necessary step toward the future of manufacturing.