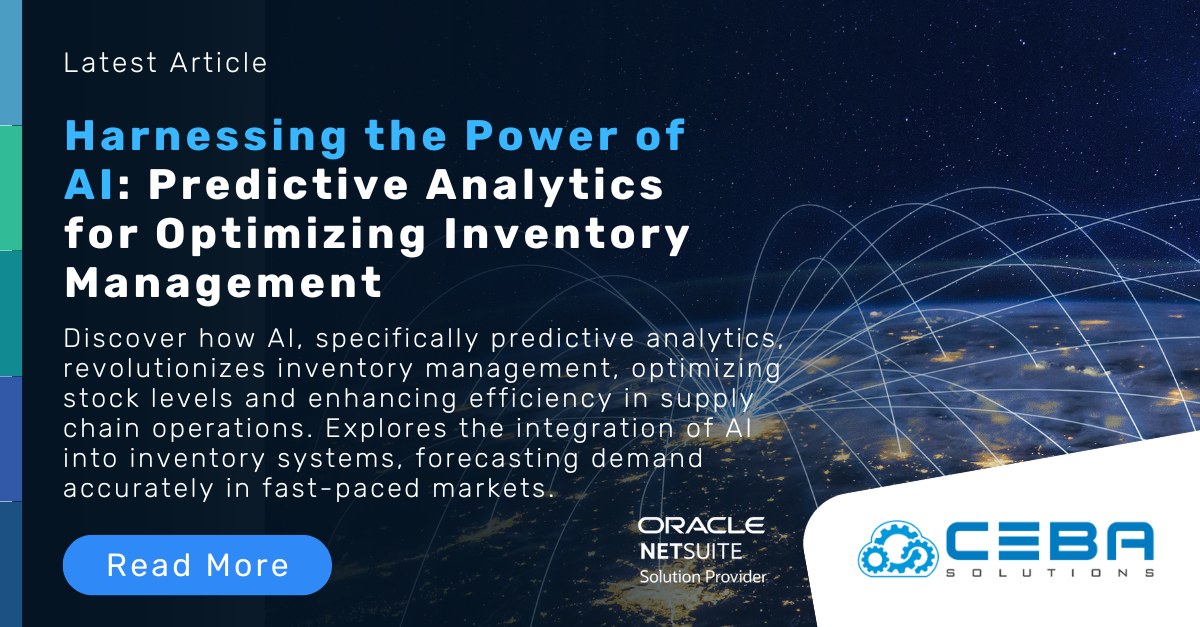
Harnessing the Power of AI: Predictive Analytics for Optimizing Inventory Management
Harnessing the Power of AI: Predictive Analytics for Optimizing Inventory Management
In today’s fast-paced and highly competitive market, efficient inventory management is crucial for the success of any business. Inventory management involves overseeing the flow of goods from manufacturers to warehouses and ultimately to the point of sale. Effective inventory management ensures that businesses maintain optimal stock levels to meet customer demand without incurring excess costs. The advent of artificial intelligence (AI) and predictive analytics has revolutionized inventory management by providing advanced tools to predict demand, optimize stock levels, and enhance overall efficiency. This article explores how businesses can harness the power of AI and predictive analytics to optimize their inventory management practices.
The Importance of Inventory Management
Effective inventory management offers several critical benefits:
Customer Satisfaction: Ensuring that products are available when customers need them enhances satisfaction and loyalty. By accurately forecasting demand and maintaining optimal stock levels, businesses can avoid disappointing customers with out-of-stock items and ensure timely fulfillment of orders. This reliability in product availability fosters trust and encourages repeat business.
Cost Efficiency: Reducing excess inventory minimizes holding costs and capital investment, while avoiding stockouts prevents lost sales. Efficient inventory management balances these two aspects, ensuring that businesses do not overinvest in inventory that ties up capital or face shortages that lead to missed revenue opportunities. Proper inventory levels also reduce storage costs and minimize the risk of obsolete stock.
Operational Efficiency: Streamlined inventory processes improve overall operational efficiency and reduce the risk of errors. By automating routine tasks and optimizing stock levels, businesses can allocate resources more effectively and ensure smooth operations across the supply chain. Efficient operations lead to faster order processing and delivery, enhancing overall productivity.
Profitability: Optimal inventory levels help maintain profit margins by balancing supply with demand. Effective inventory management prevents markdowns due to overstocking and captures sales opportunities by having the right products available at the right time. This balance between supply and demand ensures that businesses maximize their revenue potential without unnecessary expenses.
How AI and Predictive Analytics Enhance Inventory Management
AI and predictive analytics offer powerful tools to transform traditional inventory management practices. Here are some key ways they enhance inventory management:
Demand Forecasting: AI-driven predictive analytics can analyze historical sales data, market trends, and external factors to forecast future demand accurately. This helps businesses anticipate customer needs and adjust inventory levels accordingly, ensuring they have the right amount of stock on hand. Accurate demand forecasting reduces the risk of stockouts and overstocking.
Stock Level Optimization: Predictive analytics can determine optimal stock levels by analyzing factors such as lead times, sales velocity, and seasonal variations. By maintaining the right balance of inventory, businesses can minimize holding costs and reduce the risk of stockouts. This optimization ensures that resources are used efficiently and that capital is not unnecessarily tied up in inventory.
Automated Replenishment: AI can automate the replenishment process by generating purchase orders based on predicted demand and current stock levels. This reduces manual intervention, ensures timely restocking, and prevents overordering or underordering. Automated replenishment leads to a more efficient supply chain and reduces the workload on inventory managers.
Risk Mitigation: Predictive analytics can identify potential supply chain disruptions and suggest contingency plans. By analyzing supplier performance, geopolitical factors, and market conditions, AI can help businesses mitigate risks and maintain consistent inventory levels. This proactive approach helps businesses prepare for and respond to unforeseen disruptions.
Trend Analysis: AI can identify emerging trends and shifts in consumer preferences, enabling businesses to adjust their inventory strategies proactively. This helps businesses stay ahead of market changes and capitalize on new opportunities. Trend analysis ensures that businesses are responsive to changing market dynamics and customer preferences.
Inventory Segmentation: AI can segment inventory based on factors such as demand variability, product lifecycle, and profitability. This allows businesses to implement differentiated inventory strategies for different product categories, optimizing overall inventory performance. Segmentation helps businesses prioritize high-value items and manage low-demand products more effectively.
The Role of Predictive Analytics in Inventory Management
Predictive analytics involves using historical data, statistical algorithms, and machine learning techniques to forecast future outcomes. In inventory management, predictive analytics can provide valuable insights into demand patterns, stock levels, and replenishment needs. Here’s how predictive analytics can transform inventory management:
Demand Forecasting
Historical Data Analysis: Predictive models analyze historical sales data to identify patterns and trends that influence demand. This includes seasonal fluctuations, market trends, and external factors such as economic conditions and promotions. By understanding these patterns, businesses can better anticipate future demand and plan inventory accordingly. This foresight allows for more accurate inventory planning and reduces the risk of stockouts or excess inventory.
Real-Time Adjustments: Predictive analytics allows for real-time adjustments based on current sales data, enabling businesses to respond swiftly to changes in demand. This agility ensures that inventory levels remain optimal, avoiding both stockouts and excess inventory. Real-time adjustments help businesses maintain a balance between supply and demand, ensuring customer satisfaction.
Optimal Stock Levels
Safety Stock Calculation: Predictive analytics helps determine the optimal level of safety stock required to buffer against uncertainties in demand and supply. This ensures that businesses can meet customer demand without overstocking, reducing holding costs while maintaining service levels. Proper safety stock levels minimize the risk of lost sales and excess inventory costs.
Reorder Point Optimization: By forecasting future demand, predictive models can calculate the optimal reorder points for each product, ensuring timely replenishment and preventing stockouts. This minimizes the risk of running out of stock while keeping inventory levels as low as possible. Optimized reorder points ensure that inventory is replenished efficiently and cost-effectively.
Inventory Turnover Optimization
Turnover Analysis: Predictive analytics can identify slow-moving and fast-moving items, enabling businesses to optimize their inventory turnover rates. This helps in maintaining a healthy inventory flow, reducing holding costs, and freeing up capital for other uses. High turnover rates indicate efficient inventory management and effective response to market demand.
Lifecycle Management: Predictive models can forecast the lifecycle of products, helping businesses plan for product introductions, phase-outs, and discontinuations more effectively. This ensures that inventory levels are aligned with the product lifecycle, minimizing obsolescence and maximizing profitability. Lifecycle management ensures that businesses are prepared for each stage of a product's life, from launch to end-of-life.
Supplier Performance Management
Lead Time Analysis: Predictive analytics can assess supplier performance by analyzing lead times and delivery reliability. This information helps in selecting reliable suppliers and planning for potential delays, ensuring that inventory levels remain consistent and disruptions are minimized. Reliable supplier performance is crucial for maintaining smooth supply chain operations.
Order Frequency Optimization: By predicting future demand and lead times, businesses can optimize order frequencies to maintain adequate stock levels without incurring excess costs. This balance ensures that businesses have the right amount of inventory when needed, without overburdening storage facilities. Optimized order frequency reduces the risk of stockouts and minimizes storage costs.
Benefits of Predictive Analytics in Inventory Management
Enhanced Customer Satisfaction: By ensuring that products are available when customers need them, businesses can enhance customer satisfaction and loyalty, leading to repeat business and positive word-of-mouth. Satisfied customers are more likely to return and recommend the business to others.
Cost Efficiency: Predictive analytics helps reduce excess inventory and associated holding costs while preventing stockouts that can lead to lost sales. This balance improves overall cost efficiency. Efficient inventory management ensures that businesses are not overinvesting in stock or losing sales due to stock shortages.
Operational Efficiency: Streamlining inventory processes with predictive analytics reduces the risk of errors and improves overall operational efficiency, allowing businesses to focus on other strategic areas. Efficient operations lead to faster order processing and fulfillment, enhancing productivity.
Profitability: Maintaining optimal inventory levels helps businesses maintain healthy profit margins by aligning supply with demand, reducing wastage, and improving sales performance. Effective inventory management maximizes revenue potential and minimizes unnecessary costs.
Implementing Predictive Analytics for Inventory Management
Data Collection and Integration
Comprehensive Data Gathering: Collecting comprehensive data from various sources is crucial for effective predictive analytics in inventory management. This includes gathering sales records, monitoring market trends, evaluating supplier performance, and considering external factors such as economic indicators and weather conditions. By capturing a wide array of data points, businesses can build a more accurate and holistic view of their inventory needs.
Data Integration: To leverage the full potential of predictive analytics, integrating data from different systems is essential. This means unifying data from enterprise resource planning (ERP) systems, point-of-sale (POS) systems, and supply chain management platforms. Creating a cohesive dataset enables more accurate and insightful analysis, leading to better inventory management decisions.
Choosing the Right Predictive Models
Algorithm Selection: Selecting the appropriate predictive algorithms is key to successful inventory management. The choice of algorithm depends on the specific needs and characteristics of the inventory. Common algorithms include time series analysis, regression models, and advanced machine learning techniques like neural networks and decision trees. These models can forecast demand and optimize inventory levels more accurately.
Model Training and Validation: Once the predictive algorithms are chosen, they need to be trained using historical data. This involves testing the models' accuracy and refining them as necessary. Continuous evaluation and validation ensure that the predictions remain reliable and relevant over time, adapting to changing market conditions and business needs.
Integration with Inventory Management Systems
System Integration: To fully harness the benefits of predictive analytics, it's important to integrate predictive models with existing inventory management systems. This integration facilitates seamless data flow and real-time updates, ensuring that inventory decisions are based on the latest insights and trends.
Automation: Incorporating predictive insights into decision-making workflows can significantly enhance efficiency. Automating inventory management processes reduces the need for manual intervention, allowing businesses to respond more swiftly and accurately to inventory needs, thereby improving overall efficiency and reducing errors.
Continuous Monitoring and Improvement
Performance Tracking: Monitoring the performance of predictive models and inventory management processes is crucial. Key performance indicators (KPIs) such as inventory turnover, stockout rates, and holding costs provide valuable insights into the effectiveness of the predictive analytics system. Regularly tracking these KPIs helps identify areas for improvement and ensures that the system continues to deliver value.
Feedback Loop: Establishing a feedback loop is essential for maintaining the accuracy and relevance of predictive models. By continuously updating the models with new data and insights, businesses can adapt to changes in the market and improve the precision of their inventory management strategies. This ongoing refinement ensures that the predictive analytics system remains a vital tool for optimizing inventory levels and meeting customer demand effectively.
Conclusion
Harnessing the power of AI and predictive analytics for inventory management offers significant advantages for businesses seeking to optimize their operations and remain competitive in today’s market. By leveraging predictive models to forecast demand, optimize stock levels, and manage supplier performance, businesses can achieve greater efficiency, cost savings, and customer satisfaction. Implementing predictive analytics requires careful planning, data integration, and continuous improvement, but the benefits far outweigh the challenges. As technology continues to evolve, businesses that embrace predictive analytics will be well-positioned to navigate the complexities of inventory management and drive long-term success.
By integrating advanced AI and predictive analytics into their inventory management strategies, businesses can ensure they are not only meeting current market demands but also anticipating future trends and challenges. This proactive approach allows for better decision-making, increased agility, and sustained growth in an ever-changing marketplace.