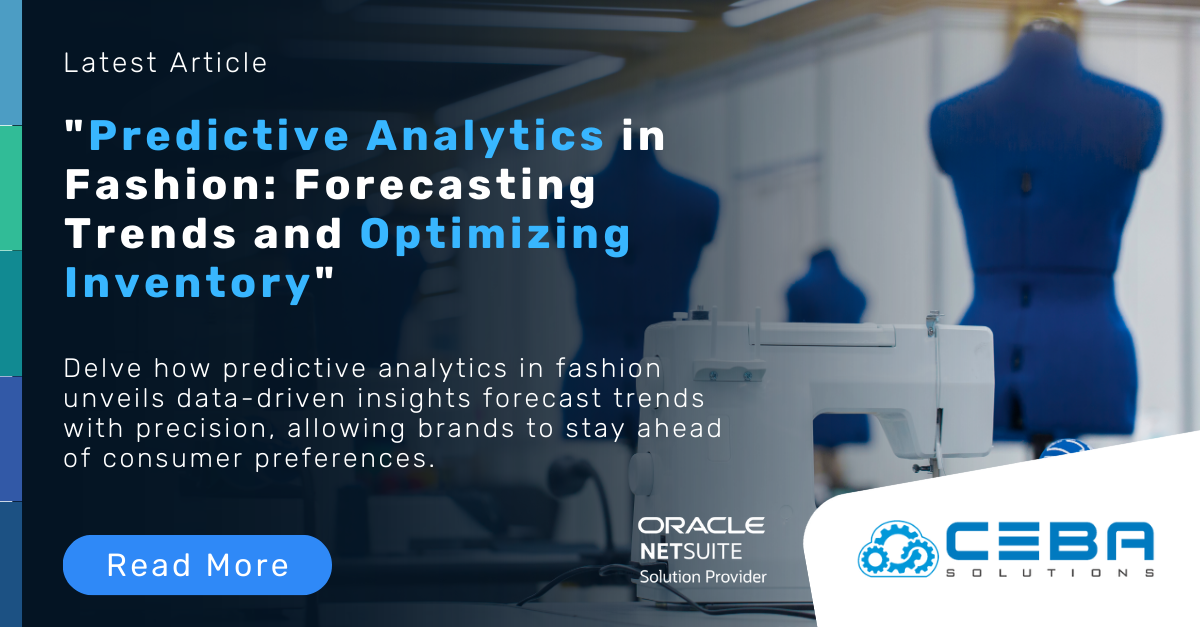
Predictive Analytics in Fashion: Forecasting Trends and Optimizing Inventory
Predictive Analytics in Fashion: Forecasting Trends and Optimizing Inventory
Predictive analytics is transforming the fashion industry by empowering brands to anticipate market trends and manage inventory with unprecedented precision. This data-driven approach leverages historical sales data, consumer behavior patterns, and broader market trends to forecast future demand, allowing fashion retailers to stay ahead of the curve. This article explores how predictive analytics is being utilized to forecast fashion trends and optimize inventory, ensuring that brands can meet consumer demands efficiently and effectively.
1. Trend Forecasting
Overview of Predictive Analytics in Trend Forecasting
Predictive analytics tools analyze vast amounts of data from various sources, including social media, online searches, past purchases, and even weather forecasts, to identify emerging fashion trends. By predicting what styles, colors, and products are likely to be popular in upcoming seasons, brands can tailor their designs to fit the anticipated market demand.
Benefits:
Proactive Design Strategy: Predictive analytics allows designers to create collections that align with predicted consumer preferences, increasing the likelihood of successful product launches. By staying ahead of trends, brands can ensure that their offerings are always relevant and appealing to their target audience. This proactive approach helps in avoiding the trial-and-error method traditionally used in fashion design.
Market Responsiveness: Brands can quickly adapt to changes in consumer tastes and stay competitive in the fast-paced fashion industry. This agility is crucial for maintaining a competitive edge and responding to real-time market dynamics. Being able to pivot quickly in response to new trends allows brands to capture market share and stay relevant.
Real-World Application:
Fashion companies like Burberry and H&M utilize predictive analytics to monitor social media trends and consumer behavior. This enables them to identify emerging trends early and incorporate them into their collections, ensuring that they remain at the forefront of fashion innovation. By analyzing patterns and sentiments from social media platforms, these brands can gauge public interest and forecast which styles will gain popularity.
2. Inventory Optimization
Overview of Predictive Analytics in Inventory Management
By accurately forecasting demand, predictive analytics helps fashion retailers determine the optimal quantity of each product to stock. This precision prevents both overstock and understock situations, reducing the risk of unsold inventory and missed sales opportunities.
Benefits:
Reduced Carrying Costs: Proper inventory levels minimize the costs associated with storing unsold goods. Efficient inventory management reduces warehousing expenses and the financial burden of excess stock. This leads to significant cost savings and improves the overall profitability of the business.
Increased Sales Potential: Adequate stock levels ensure that customer demand is met without the risk of stockouts, maximizing sales opportunities. This balance enhances customer satisfaction and loyalty by providing consistent product availability. Satisfied customers are more likely to return, boosting repeat business and long-term revenue.
Real-World Application:
Retail giants like Zara employ predictive analytics to optimize their inventory. By analyzing sales data and market trends, they can maintain optimal stock levels, reducing waste and ensuring that popular items are always available to customers. Zara’s ability to predict demand accurately and adjust inventory in real-time allows the company to respond swiftly to market changes, maintaining its position as a leader in fast fashion.
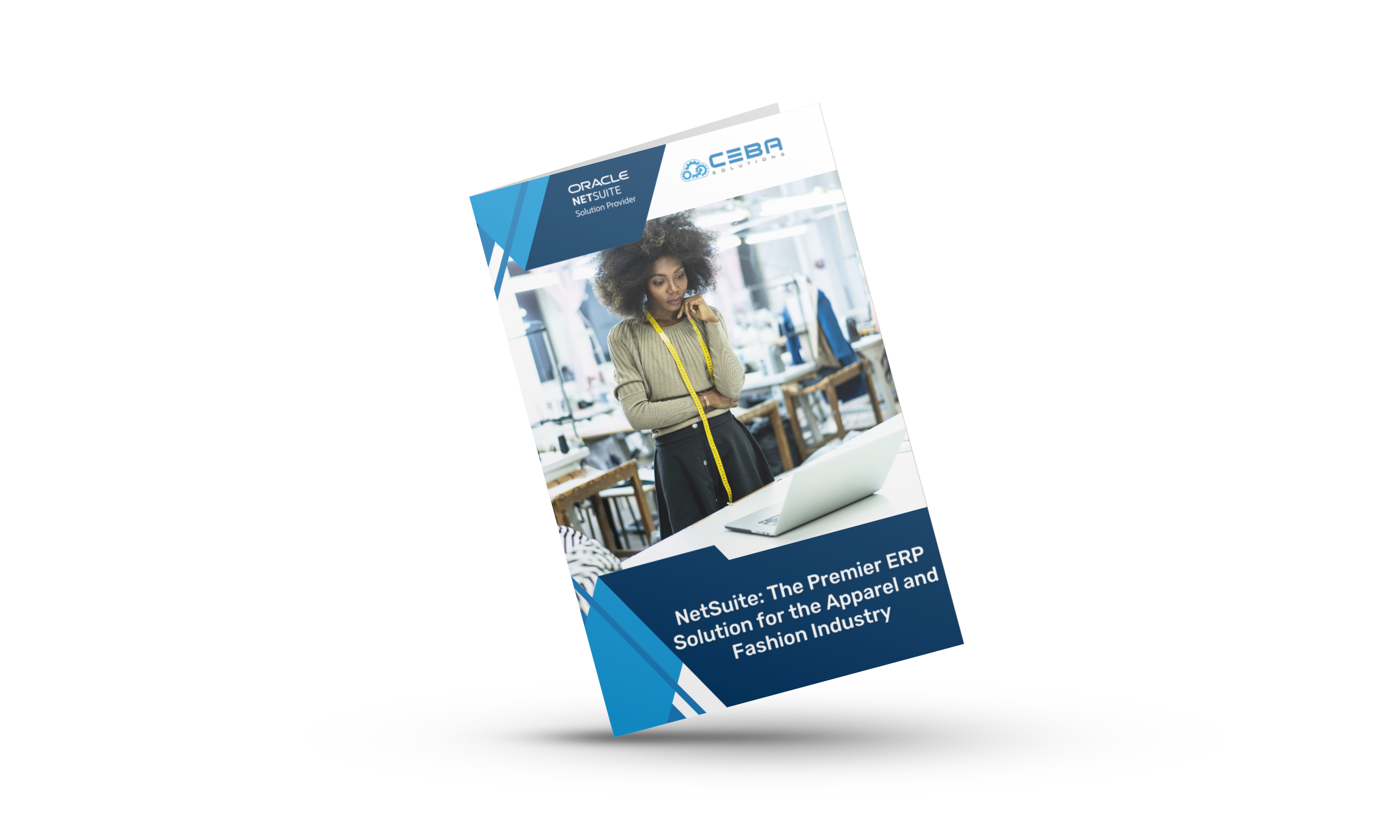
3. Dynamic Pricing
Overview of Dynamic Pricing
Predictive analytics also enables dynamic pricing strategies by assessing demand trends, competitor pricing, and inventory levels in real-time. This approach allows fashion retailers to adjust prices dynamically to maximize profits, clear out inventory, or respond to competitive pressures.
Benefits:
Profit Maximization: Optimal pricing strategies enhance profitability on each item sold. By dynamically adjusting prices based on demand, retailers can increase their margins and revenue. This data-driven approach ensures that prices are set at the most advantageous levels, reflecting real-time market conditions.
Market Competitiveness: Keeps pricing competitive based on real-time market conditions and consumer demand. This responsiveness helps retailers attract price-sensitive customers and maintain market share. By staying agile, brands can quickly respond to competitor pricing strategies and market fluctuations.
Real-World Application:
Amazon uses predictive analytics for dynamic pricing, adjusting prices multiple times a day based on a variety of factors. This approach helps them stay competitive and maximize profits by aligning prices with current market conditions and consumer behavior. By using dynamic pricing, Amazon can offer attractive deals to customers while optimizing their revenue.
4. Customer Segmentation and Personalization
Overview of Customer Segmentation
Advanced predictive models segment consumers based on purchasing behavior, preferences, and demographic data. This segmentation allows fashion brands to tailor marketing efforts and product recommendations to specific groups, enhancing the effectiveness of promotional campaigns and improving customer satisfaction.
Benefits:
Targeted Marketing: Personalized marketing messages resonate more deeply with consumers, increasing engagement and conversion rates. By targeting specific segments with tailored content, brands can achieve higher marketing ROI. This precision in marketing helps in crafting messages that directly address the needs and preferences of each segment.
Enhanced Customer Experience: By understanding and catering to individual preferences, brands can offer a more personalized shopping experience, boosting customer loyalty. Personalized recommendations and promotions make customers feel valued and understood, leading to higher satisfaction and repeat business.
Real-World Application:
Netflix-style recommendation engines are now being used by fashion retailers like Stitch Fix. These platforms analyze customer preferences and purchase history to provide personalized product recommendations, significantly enhancing the shopping experience. By leveraging predictive analytics, Stitch Fix can deliver curated fashion items that match individual tastes, improving customer retention and satisfaction.
5. Supply Chain Management
Overview of Predictive Analytics in Supply Chain
Predictive analytics improves supply chain management by forecasting potential disruptions and suggesting optimal responses. This capability helps fashion brands manage their supply chains more proactively, ensuring smooth operations even in the face of uncertainties.
Benefits:
Supply Chain Resilience: Enhanced forecasting abilities allow brands to anticipate and mitigate potential supply chain disruptions. Proactive management reduces the impact of unforeseen events on production and delivery schedules. This resilience ensures that the supply chain remains robust even during crises.
Operational Efficiency: Optimized supply chain operations reduce costs and improve the overall efficiency of the business. By aligning supply chain activities with predicted demand, brands can streamline operations and reduce lead times. This efficiency translates to better resource utilization and lower operational costs.
Real-World Application:
Nike uses predictive analytics to manage its global supply chain. By forecasting demand and potential disruptions, Nike can adjust its production schedules and logistics plans, ensuring that products are delivered on time and in the right quantities. This proactive approach allows Nike to maintain high standards of service and operational efficiency.
Conclusion
Predictive analytics is a powerful tool in the modern fashion industry, enabling brands to forecast trends, optimize inventory, and refine pricing strategies effectively. As this technology continues to evolve, its role in driving innovation and efficiency in fashion will only grow, helping brands stay relevant and competitive in a rapidly changing market.
The adoption of predictive analytics allows fashion retailers to make data-driven decisions that enhance customer satisfaction, improve operational efficiency, and drive profitability. As the fashion industry continues to embrace digital transformation, predictive analytics will be at the forefront, shaping the future of how brands understand and meet consumer demands.
By integrating predictive analytics into their operations, fashion brands can anticipate market trends, optimize inventory levels, and implement dynamic pricing strategies that maximize profitability. This data-driven approach not only improves business outcomes but also enhances the overall customer experience, ensuring that fashion brands can thrive in a competitive marketplace. The future of fashion is data-driven, and predictive analytics is leading the way in this transformation, offering new opportunities for innovation and growth in the industry.