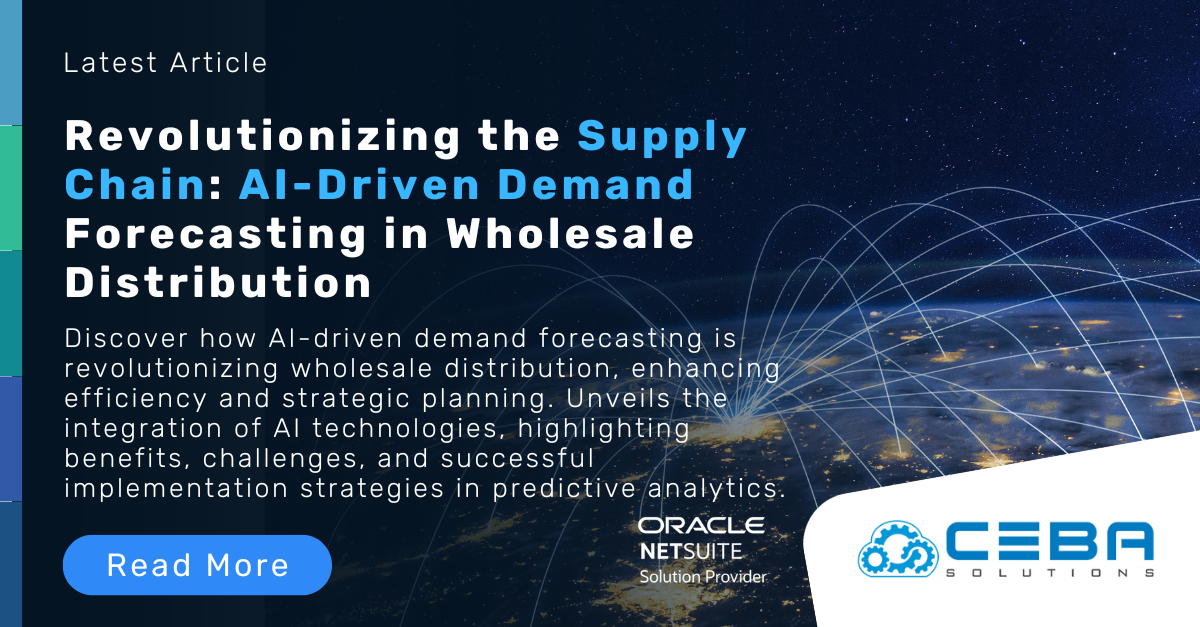
Revolutionizing the Supply Chain: AI-Driven Demand Forecasting in Wholesale Distribution
Revolutionizing the Supply Chain: AI-Driven Demand Forecasting in Wholesale Distribution
In today's fast-paced business environment, wholesale distributors face mounting pressure to maintain competitiveness and profitability. At the heart of this challenge lies the critical task of supply chain management, with demand forecasting playing a pivotal role. Traditional forecasting methods, often reliant on historical data and manual adjustments, struggle to keep pace with the complexities of modern markets. Enter artificial intelligence (AI)-driven demand forecasting—a game-changing approach that harnesses the power of advanced algorithms and machine learning to transform supply chain management.
The Crucial Role of Demand Forecasting in Wholesale Distribution
Accurate demand forecasting is not just a nice-to-have; it's a fundamental necessity for wholesale distributors. Here's why:
Inventory Optimization: Precise forecasting ensures that wholesalers maintain optimal stock levels, striking the delicate balance between meeting customer demand and avoiding excess inventory. By accurately predicting future demand, wholesalers can adjust their inventory levels in real-time, reducing the risk of overstocking slow-moving items or understocking popular products. This optimization leads to improved cash flow and reduced carrying costs, allowing businesses to allocate resources more efficiently.
Cost Reduction: By minimizing overstocking and understocking scenarios, wholesalers can significantly reduce costs associated with storage, obsolescence, and emergency shipments. Accurate forecasting allows for more efficient use of warehouse space, reduces the need for clearance sales on excess stock, and minimizes the need for expensive rush orders to meet unexpected demand spikes. These cost savings can directly impact the bottom line and improve overall profitability, enhancing the financial health of the business.
Customer Satisfaction: Reliable product availability is key to customer retention and loyalty. Accurate forecasting helps ensure that products are available when and where customers need them. This reliability builds trust and can lead to increased customer loyalty and repeat business. In a competitive market, the ability to consistently meet customer demands can be a significant differentiator, helping wholesalers maintain strong relationships with their retail partners.
Operational Efficiency: Forecasting impacts every aspect of the supply chain. Accurate predictions enable streamlined procurement, production scheduling, and distribution processes. With better forecasts, wholesalers can optimize their entire operation, from negotiating better terms with suppliers based on more accurate order quantities to improving labor scheduling in warehouses. This end-to-end efficiency can lead to significant improvements in overall operational performance, making the supply chain more agile and responsive.
The Mechanics of AI-Driven Demand Forecasting
AI-driven demand forecasting represents a quantum leap from traditional methods. Here's a closer look at how it works:
Comprehensive Data Gathering: AI systems cast a wide net, collecting data from diverse sources. This includes historical sales data, market trends, economic indicators, seasonal patterns, and even external factors like weather conditions and social media trends. By incorporating a broad range of data points, AI-driven forecasting can capture nuances and influences that might be missed by more limited traditional methods. For example, it might identify correlations between social media sentiment and demand for certain products, or between weather patterns and seasonal item sales.
Data Integration: The power of AI lies in its ability to integrate data from various systems, including ERP, CRM, and POS systems, creating a unified, rich dataset for analysis. This integration allows for a holistic view of the business, breaking down silos that often exist in traditional forecasting methods. By combining data from multiple sources, AI can identify complex relationships and dependencies that might not be apparent when looking at each data source in isolation.
Pattern Recognition: Advanced machine learning algorithms sift through vast amounts of data, identifying complex patterns and correlations that human analysts might miss. These algorithms can detect subtle trends and cyclical patterns that may be imperceptible to the human eye, especially when dealing with large volumes of data across multiple product lines and markets. This capability allows businesses to understand their demand drivers better and make more informed decisions.
Predictive Modeling: Using sophisticated techniques such as regression analysis, time series forecasting, and neural networks, AI creates predictive models that forecast future demand with unprecedented accuracy. These models can adapt and learn over time, continuously improving their accuracy as they are exposed to more data and outcomes. This dynamic learning capability allows AI-driven forecasting to outperform static traditional models, especially in volatile or rapidly changing markets.
Dynamic Forecasting: Unlike static traditional models, AI-driven forecasts are continuously updated in real-time as new data becomes available. This allows for rapid adjustments to changing market conditions and customer behaviors. For example, if a sudden trend emerges on social media that impacts demand for a particular product, an AI-driven system can quickly adjust its forecast and alert the wholesaler to potential inventory needs, ensuring that supply can meet demand.
Anomaly Detection: AI excels at detecting anomalies and outliers in data, enabling quick responses to unexpected events or demand spikes. This capability is particularly valuable in identifying potential supply chain disruptions or sudden shifts in consumer behavior. By flagging these anomalies early, wholesalers can take proactive measures to mitigate risks or capitalize on opportunities, maintaining smooth operations even in the face of unpredictability.
The Multifaceted Benefits of AI-Driven Demand Forecasting
The advantages of implementing AI-driven demand forecasting are numerous and significant:
Improved Accuracy
Data-Driven Insights: By relying on comprehensive data analysis rather than human intuition, AI-driven forecasting dramatically improves prediction accuracy. This data-centric approach minimizes biases and subjective judgments that can skew traditional forecasting methods. The result is a more reliable and consistent forecast that can be trusted to guide critical business decisions, enhancing strategic planning and operational execution.
Enhanced Precision: AI models can consider a multitude of factors and complex relationships simultaneously, leading to more nuanced and accurate forecasts. This level of complexity is beyond the capability of human analysts or traditional statistical models. For example, an AI model might simultaneously consider historical sales data, current economic indicators, weather forecasts, and social media trends to predict demand for a specific product in a particular region.
Cost Savings
Inventory Reduction: Accurate forecasting helps wholesalers maintain lean inventories, reducing holding costs and minimizing waste from obsolete stock. By precisely predicting demand, wholesalers can avoid tying up capital in excess inventory while still ensuring they have sufficient stock to meet customer needs. This optimization can lead to significant savings in warehouse costs, insurance, and inventory depreciation, freeing up capital for other business initiatives.
Efficient Resource Allocation: By optimizing inventory levels and procurement schedules, wholesalers can avoid costs associated with stockouts, overstocking, and rush orders. This efficiency extends beyond inventory to impact other areas of the business, such as labor scheduling and transportation planning. With more accurate forecasts, wholesalers can allocate their resources more effectively across the entire supply chain, ensuring that all operations are running at optimal levels.
Enhanced Customer Satisfaction
Reliable Availability: Ensuring consistent product availability builds customer trust and loyalty. When customers can rely on a wholesaler to have the products they need when they need them, it strengthens the business relationship and can lead to increased order volumes and long-term partnerships. This reliability is crucial in maintaining a competitive edge in the market.
Reduced Stockouts: By minimizing stockouts, wholesalers can prevent lost sales and maintain a positive customer experience. Stockouts not only result in immediate lost revenue but can also damage customer relationships and lead to long-term loss of business. AI-driven forecasting helps mitigate this risk by more accurately predicting demand and ensuring adequate stock levels, maintaining customer satisfaction and trust.
Operational Efficiency
Streamlined Processes: Accurate forecasts enable better planning and coordination across the entire supply chain, from procurement to distribution. This improved coordination can lead to more efficient operations, reduced lead times, and better utilization of resources. For example, more accurate forecasts can help optimize production schedules, reducing changeover times and improving overall equipment effectiveness.
Proactive Management: AI-driven insights allow wholesalers to anticipate and proactively manage supply chain disruptions and demand fluctuations. Rather than reacting to changes after they occur, wholesalers can take preemptive action to mitigate risks or capitalize on opportunities. This proactive approach can be a significant competitive advantage in dynamic markets, allowing businesses to stay ahead of trends and maintain smooth operations.
Implementing AI-Driven Demand Forecasting: A Roadmap to Success
While the benefits are clear, implementing AI-driven demand forecasting requires careful planning and execution. Here's a roadmap for successful implementation:
Data Preparation: Ensuring data quality is the foundation of successful AI-driven forecasting. This process involves cleaning and preprocessing data to handle missing values, outliers, and inconsistencies. It's crucial to establish data governance protocols to maintain data integrity over time. This may involve implementing data validation checks, establishing data entry standards, and regularly auditing data sources. Creating a unified dataset by integrating data from various sources is equally important. This may require developing data pipelines to automatically collect and consolidate data from different systems. The goal is to create a single source of truth that the AI model can rely on for accurate forecasting.
Algorithm Selection: Choosing appropriate machine learning models based on specific forecasting needs and data characteristics is a critical step. This may involve experimenting with different algorithms such as ARIMA (AutoRegressive Integrated Moving Average), Prophet, or deep learning models like LSTM (Long Short-Term Memory) networks. The choice of algorithm will depend on factors such as the complexity of the demand patterns, the amount of historical data available, and the specific requirements of the business. Training and validating models using historical data is an iterative process. It involves splitting the data into training and testing sets, evaluating model performance using appropriate metrics (e.g., Mean Absolute Error, Root Mean Square Error), and refining the models to optimize their performance. This process may also include feature engineering to create new variables that can improve the model's predictive power.
System Integration: Seamlessly integrating AI forecasting models with existing ERP, CRM, and inventory management systems is crucial for realizing the full benefits of AI-driven forecasting. This integration ensures that the forecasts are readily available to all relevant stakeholders and can be automatically fed into other business processes. It may require developing APIs or using middleware solutions to facilitate data exchange between systems. Developing user-friendly interfaces is equally important to ensure that business users can easily interact with forecasting models and gain actionable insights. These interfaces should provide intuitive visualizations of forecasts, allow for scenario analysis, and enable users to provide feedback or manual adjustments when necessary. The goal is to make the power of AI accessible to non-technical users who make critical business decisions.
Continuous Monitoring and Improvement: Tracking the performance of AI forecasting models using key performance indicators (KPIs) such as forecast accuracy and inventory turnover is essential for maintaining the system's effectiveness over time. This may involve setting up automated reporting systems that regularly compare forecasts to actual demand and highlight any significant deviations. Continuously updating and refining models with new data and feedback is crucial to maintain accuracy and relevance. As market conditions change and new data becomes available, the models should be retrained and fine-tuned. This may also involve periodically reassessing the choice of algorithms and features to ensure they remain optimal for the current business environment.
Change Management and Training: Securing buy-in from key stakeholders across the organization is critical for successful adoption of AI-driven forecasting. This involves clearly communicating the benefits of the new system, addressing concerns, and involving stakeholders in the implementation process. It may be helpful to start with a pilot project to demonstrate the value of AI-driven forecasting before rolling it out across the entire organization. Providing comprehensive training programs helps staff understand and effectively use AI tools and insights. This training should cover not only the technical aspects of using the new system but also the principles of data-driven decision making. It's important to foster a culture that values data and analytics, encouraging employees to base their decisions on insights provided by the AI system rather than relying solely on intuition or past practices.
The Future of AI-Driven Demand Forecasting
As technology continues to evolve, several trends are shaping the future of AI-driven demand forecasting:
Integration with IoT and Big Data: Leveraging data from IoT devices will provide real-time insights into inventory levels and supply chain conditions. For example, smart shelves in retail stores could automatically update inventory levels, providing wholesalers with instant visibility into stock depletion rates. Connected vehicles and shipping containers could provide real-time data on the location and condition of goods in transit, allowing for more accurate delivery time predictions. Advanced big data analytics techniques will enhance the ability to process and analyze massive datasets. This includes the use of distributed computing frameworks like Apache Spark for processing large volumes of data in real-time, and the application of advanced techniques like deep learning on unstructured data sources such as images and text.
AI-Powered Supply Chain Platforms: End-to-end AI solutions will offer comprehensive visibility and optimization across the entire supply chain. These platforms will integrate demand forecasting with other critical functions such as procurement, production planning, and logistics optimization. By considering the entire supply chain holistically, these platforms can identify opportunities for efficiency and cost savings that might be missed when optimizing each function in isolation. Collaborative forecasting and planning across the supply chain will become more prevalent, fostering greater coordination and efficiency among suppliers, manufacturers, and distributors. AI will facilitate the sharing of relevant data and insights among supply chain partners, enabling more accurate forecasts and better-aligned operations across the entire value chain.
Final Thoughts
AI-driven demand forecasting represents a paradigm shift in supply chain management for wholesale distributors. By leveraging advanced machine learning algorithms and real-time data analysis, businesses can achieve unprecedented levels of accuracy in predicting customer demand. This leads to optimized inventory levels, reduced costs, improved customer satisfaction, and enhanced operational efficiency.
While implementing AI-driven forecasting requires careful planning and execution, the benefits far outweigh the challenges. As technology continues to evolve, AI-driven demand forecasting will play an increasingly vital role in navigating the complexities of modern supply chains and driving long-term success in the wholesale distribution industry.
The future of wholesale distribution belongs to those who can harness the power of AI to turn data into actionable insights. By embracing AI-driven demand forecasting, wholesalers can position themselves at the forefront of their industry, ready to meet the challenges and opportunities of an ever-changing market landscape.